In the context of the geophysics and seismic data processing, the pursuit of accuracy and efficiency has driven the application of cutting-edge technologies, such as deep learning. One of the most exciting applications of semblance features and deep learning is improving accuracy of velocity models. This blog discusses how these are interdependent, and what this means for seismic interpretation and exploring the subsurface. This blog describes about emblance features deep learning velocity model.
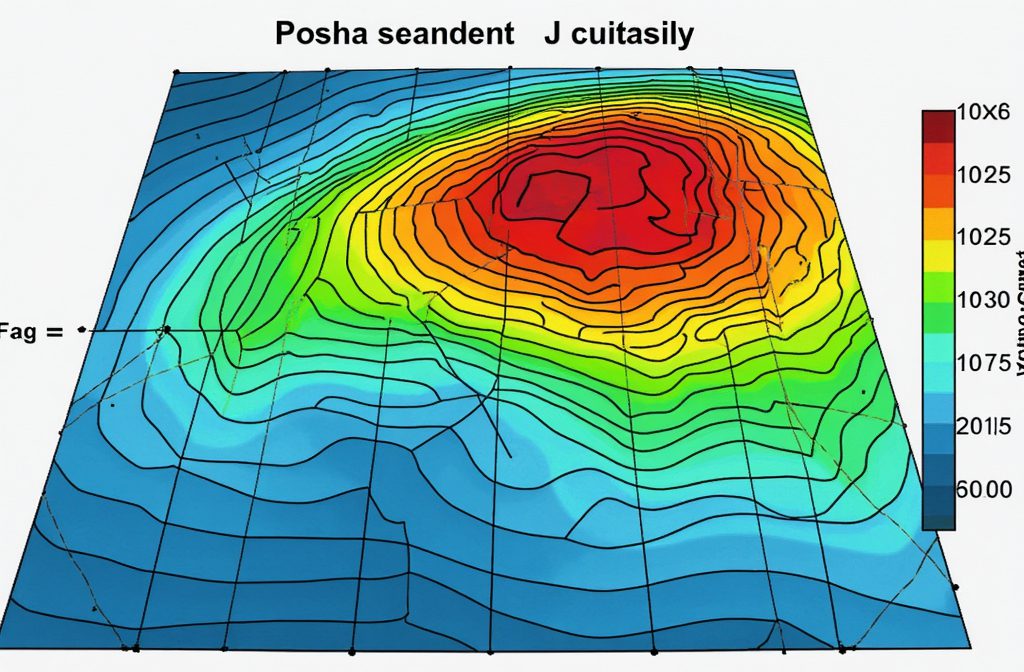
Semblance Features
Semblance attributes are the interpretation workhorse for seismic imaging, and provide interpretation into subsurface structure. These are measuring the legitimacy of the seismic signals from traces, where reflections are consistently and align well. This continuity is important to determine geological boundaries, including layers, faults and folds. Semblance features deep learning velocity model
Important properties of semblance features:
- Measurement of Coherence: Semblance analyses the similarity of seismic signals and helps in recognizing continuous geological structures.
- Visualization: Anton can generate a graphic representation of the Earth’s depth below subsurface, to facilitate reading of seismic data by geophysicists.
- Semblance Attributes: Semblance values provide fundamental input into the construction of accurate velocity models, they reveal the distribution of geological horizons.
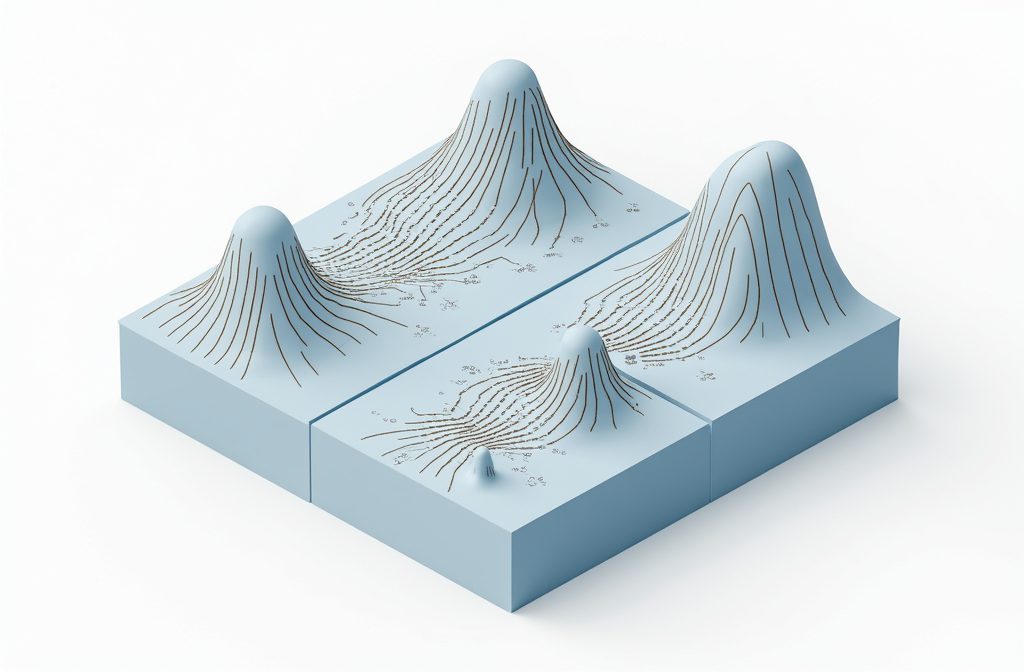
The Importance and Role of Velocity Models in Seismic Inversion
Velocity models are special tools used to compensate for the time delay impacts of the subsurface rocks. They form the theoretical basis for interpreting the subsurface of the Earth, for imaging it and for exploring its resources. Semblance features deep learning velocity model
Significance of Velocity Models:
- Tracking Seismic Wavefields: Seismic images benefit from velocity models, through which seismic reflections can be mapped to corresponding geological bodies.
- Resource Discovery: They are essential for finding oil, gas and minerals.
- Reducing risk: By accurately predicting the velocity of seismic waves, the researchers can estimate geological hazards, such as earthquakes and landslides.
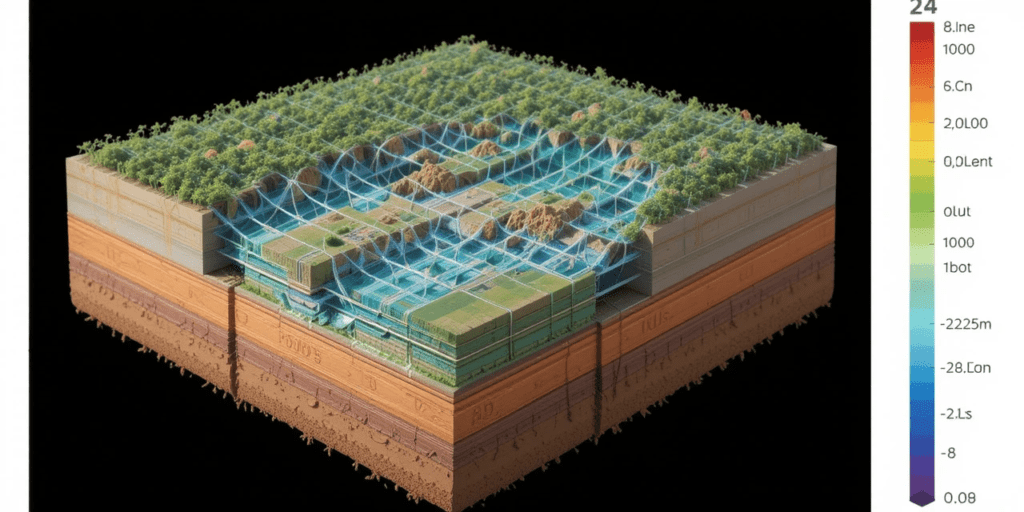
Deep learning velocities – A game changer in velocity model building
A branch of artificial intelligence, deep learning, has brought a paradigm shift in the seismic data analysis by automating challenging tasks and discovering subtle patterns. Its application in VMB has sparked new opportunities for both precision and efficiency.
Deep Learning Contributions to Velocity Models:
- Process Data: Through the use of deep learning algorithms, students are able to process large volumes of data, revealing useful patterns within seismic signals.
- Pattern detection: Neural networks detect subtle semblance features that other methods may fail to recognize.
- Automation: Tasks such as velocity picking and model updating are automated thereby reducing manual labour and errors.
- Flexibility: The trained deep learning models work on a range of data, making them adaptable to different geological conditions.
The Interplay of Semblance and Deep Learning
The marriage between semblance features and deep learning has become a powerful driver for improving the accuracy of velocity models. This cooperative work serves to benefit both methods and provides more consistent and comprehensive subsurface interpretations.
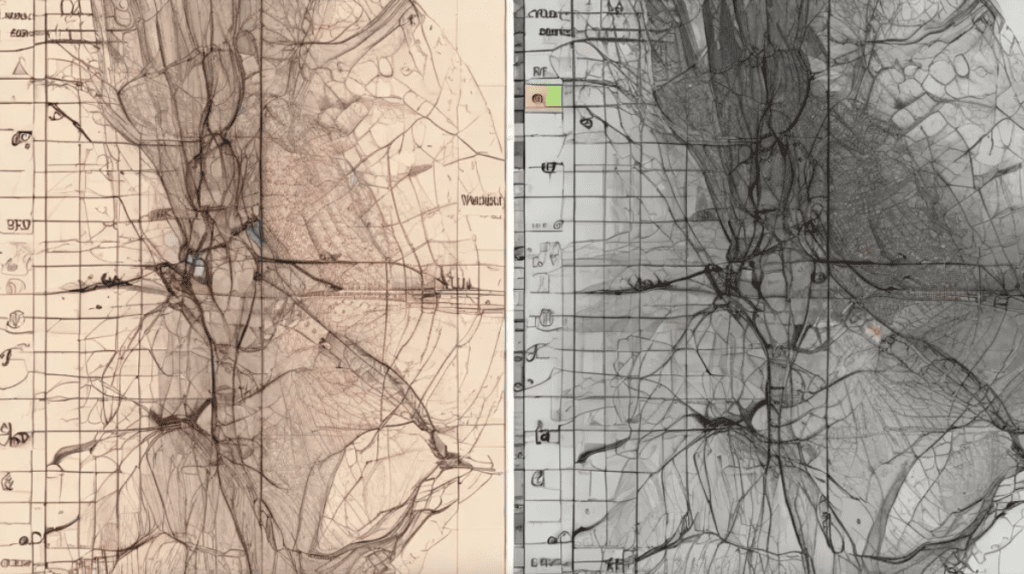
Advantages of Integrating Semblance Features and Deep Learning:
- Increased Precision: Deep learning models make semblance features more precise to refine velocity models.
- Efficient: Automatic analysis speeds up the velocity model building process, elimininating time and costs.
- Scalability: The technique is compatible with largescale seismic surveys, and expansive exploration area projects can be pursued.
Applications in the Field
The applications of semblance features and deep learning in velocity model building are enormous including oil and gas exploration, and environmental studies.
Case Studies:
- Oil and Gas: The industry uses this technology to aid in finding hydrocarbon deposits with more precision.
- Seismology: Velocity models help us to understand the dynamics of faults and to forecast seismicity.
- Environmental Monitoring: They are used to evaluate subsoil conditions for construction purposes and to study groundwater.
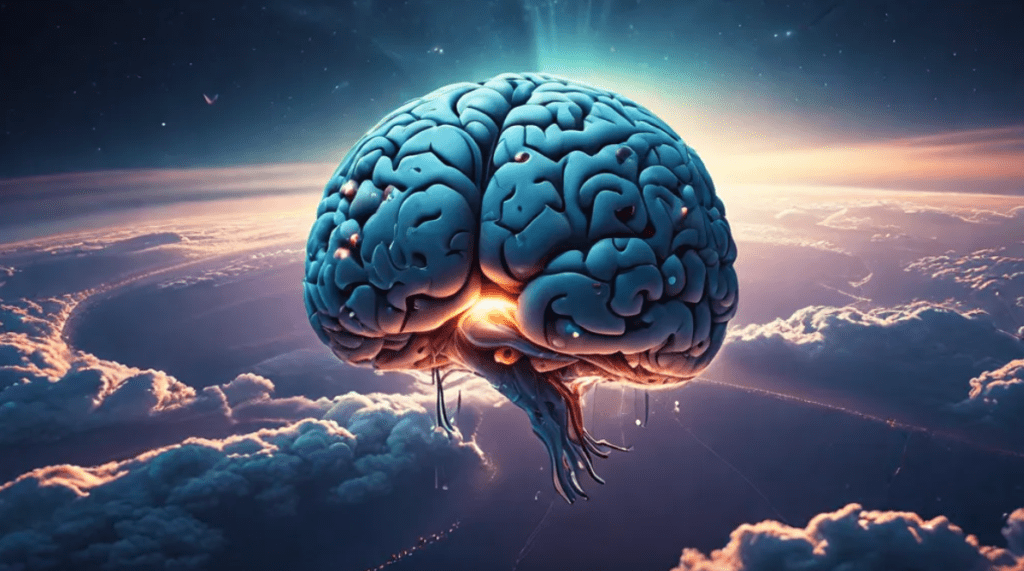
Challenges and perspectives
Despite great promise of semblance features and deep learning integration it is not free of challenges. These problems must be faced in order to obtain the advantages of the method.
Challenges:
- Data quality: The quality of seismic data can influence on the accuracy of velocity models through noise content and inconsistency.
- Computational Needs: Deep learning models are computational intensive.
- Complexity of Predictions: Deep learning-based predictions may be complex and require experts for interpretation.
Future Directions:
- Advanced Algorithms: Researching on advanced computer aided algorithms for complex geology resins with advanced patterns deep learning.
- RTA: Speeding-up the data access for real-time seismological investigation.
- Integration with Other Technologies: Incorporation of deep learning with others types of AI, such as reinforcement learning, for richer outcomes.
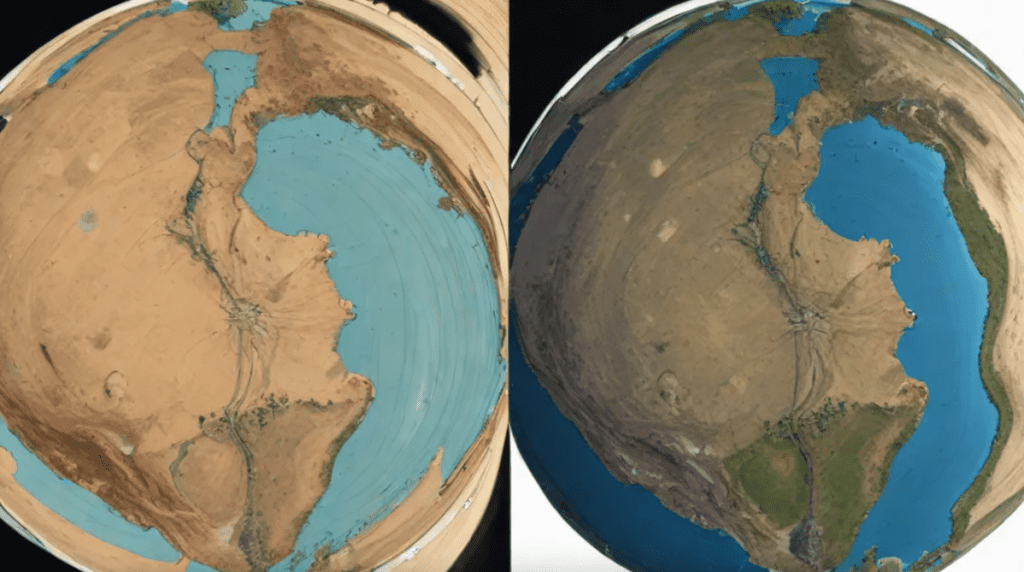
Practical Applications of Deep Learning in Velocity Model:
In this paper, we demonstrate, by means of some representative results, that deep learning can actually be put into use for velocity models, by integrating with data preprocessing and algorithm training, and can even be integrated with seismic analysis workflow. Here is a step-by-step guide to how it works:
Data Preparation
- High-quality input data is requisite in deep learning to achieve correct results. For velocity models that means The same holds true for velocity models :
- Collection of seismic data: Collect raw seismic data from surveys, whose value is recorded in waveforms and semblance attributes.
- Preprocessing: Purification and denoising of the data. Pre-processing methods such as filtering and normalization rescale the seismic signals for deep learning.
- Annotation: Label a dataset with geological features, or the value of the velocities, in order to effectively train the model.
Deep Learning Model Training
Here, the practical aspects are centered on the training of the deep learning model:
- Model Selection: Select an appropriate architecture for the task like CNNs for spatial data and RNNs for sequential data.
- Feature Selection: employ semblance features together with other seismic attributes as model’s inputs. These are the features that help the algorithm to recognize patterns in the velocity differences.
- Supervised Learning: You train the model with labeled data, so it learns how to predict velocity values, or geological structures.
- Validation and Testing: Divide the data into training, validation and testing data sets to determine how accurate and well the model performs.
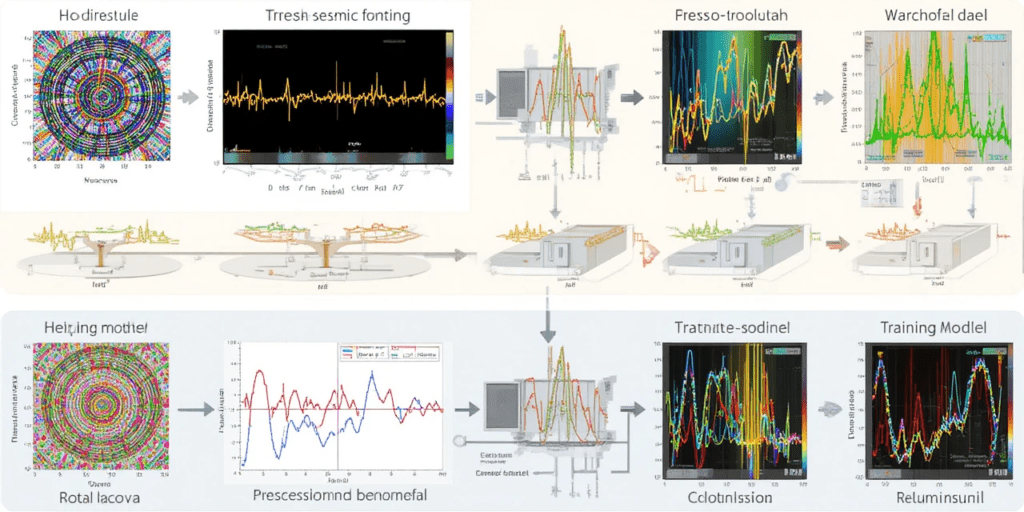
Seismic Workflow Integration
After it is trained, the deep learning model can be deployed to those who need the technology in their real-life workflow:
- Velocity Picking: Automatically pic velocities based da semblance features to save geophysicists’ time and effort.
- Refinement: Iteratively refine the velocity model using predictions of the deep network.
- Imaging improvement: Use velocity model for seismic imaging methods (migration) to get sharper sub-surface model.
- Real-Time Model: Implement constitution of the model in a system with real time computation during seismic surveys.
Advantages of Practical Application
Efficiency Reduce the manual processing work and enhance the processing speed of the velocity model.
- Consistency: Guarantee the same velocity pick and interpretation across different datasets.
- Higher Accuracy: It increases the accuracy of velocity models which results in better imaging and exploration of the subsurface.
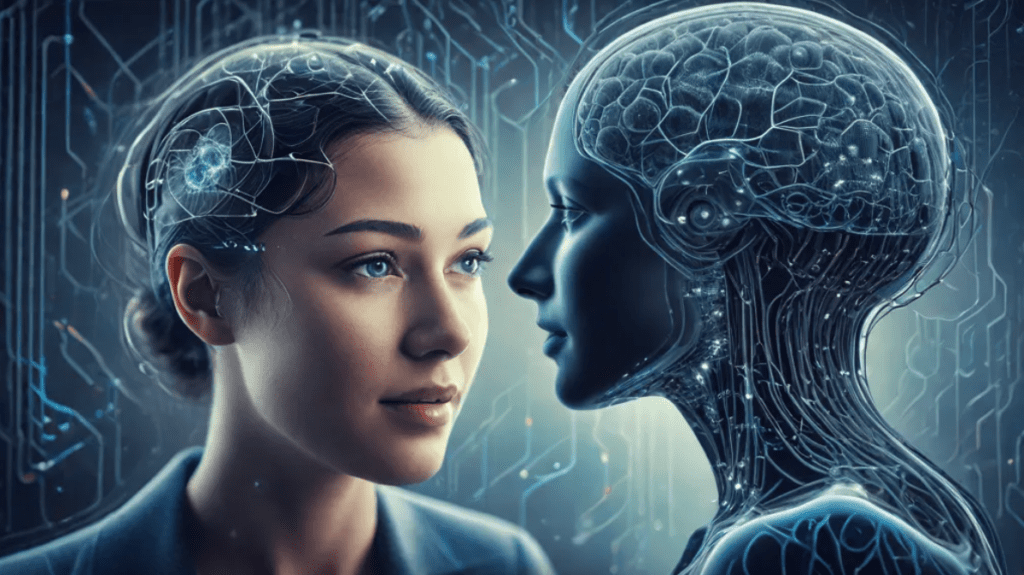
Challenges to Address
There are also some practical obstacles that need to be overcome:
- Quality of the Data : Low-quality seismic data can have an impact on the accuracy of the model predictions.
- Computational Assets: Deep learning needs powerful hardware in order to manipulate big data.
- Expertise: The training and deployment of models require expertise from individuals with backgrounds in both geophysics and AI.
Deep learning is proving to be the new driving force in velocity model building, and their evidence in being able to help geophysicists, to better understand the details of the subsurface. If you’d like a deep dive on any specific part of the implementation, drop me a line!
What are the obstacles to adopting this technology?
It is challenging to adopt deep learning for velocity model quality. These obstacles are both technical, logistical, and human challenges. Here is a deeper look at the major obstacles:
Data Quality and Availability
- Noise in Seismic Data: Seismic data may involve noise and artifacts which tend to degrade semblance events, affect the efficacy of deep learning model.
- Unstructured Training Data: Differences among the data formats, resolutions, and acquisition methods prevent the designing of uniform training datasets.
- Lack of Labeled Data: Deep learning is data hungry method and needs a large an- notated data, the labeled seismic data are generally limited, this is because of the time and experienced labor needed for manual annotation.
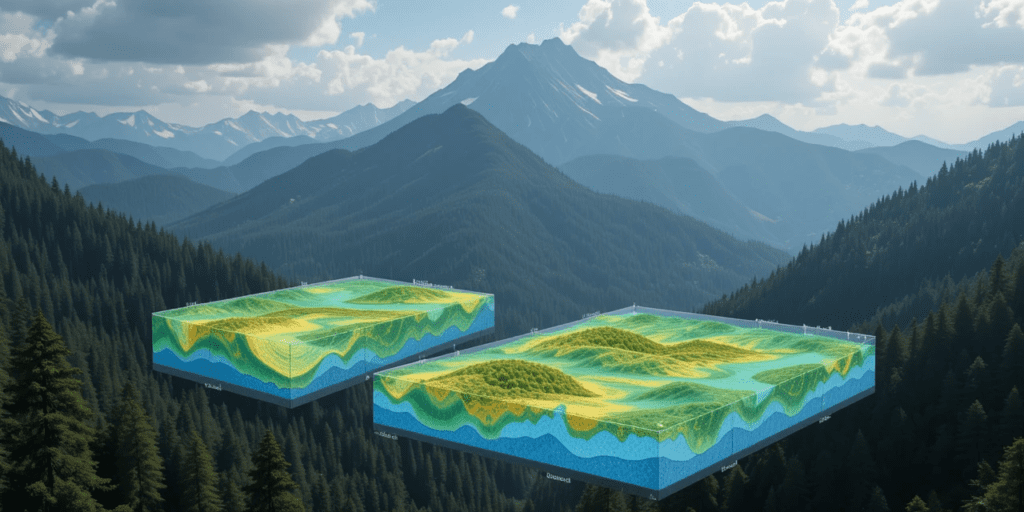
Computational Resources
- BP1: High processing power: Training deep learning models requires high computational resources such as GPU and cloud processing.
- Storage Capacity: Seismic data volumes are huge which mandates huge storage capacity for efficient processing and training of deep learning algorithms.
- Energy: Deep learning is energy hungry, which has environmental and financial implications due to constant models retraining and modifications.
Algorithm Limitations
- Model Interpretability: Neural networks are referred to as “black boxes”, causing complications to interpret how decisions are made, leading to a lack of trust in the model’s prediction.
- Overfitting: Models trained on a local dataset well might work well on similar data but poorly on new geological domains.
- Algorithmic Bias: If the training data are biased or incomplete, then the obtained velocity models can have a bias. This may lead misinterpreted subsurface information.
Skill Gap and Expertise
- Specialized Knowledge: Skills at deploying and managing deep learning models for geophysics, and skills in AI, are difficult skills to find together.
- Training Requirements: AI for geophysicists and engineers is required and there is a learning curve.
- Resistant to Change: Traditional players in the industry may be skeptical to replace standard processes with AI.
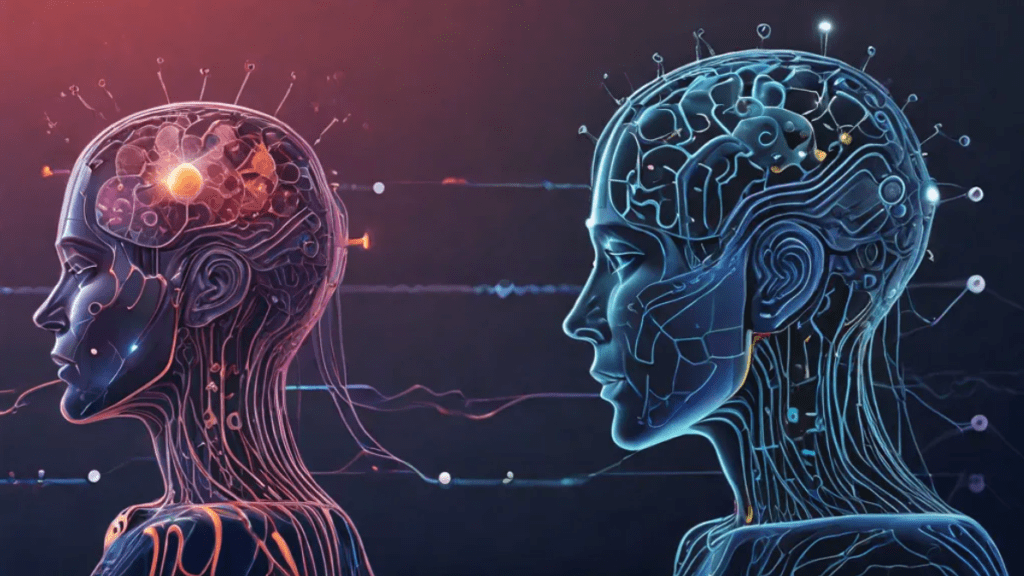
Integration Challenges
- Workflow Compatibility: Integration of deep learning into established seismic analysis workflows introduces adaptation challenges and potential operating disturbances at the beginning.
- Interoperability: It can take time and effort to integrate AI tools into your existing hardware and software.
- Practical Application: It is difficult to achieve throughput RTVM updating for the Seismic Survey case due to how fast and how accurate such an update must take place.
Ethical and Legal Issues
- Data Privacy: Seismic data, particularly private stage data, are sensitive and adhering to the data privacy requirements must be respected.
- Ethical AI Use: Issues of fairness, accountability, and transparency of AI models in geophysics at the forefront need the guidance or ethical principles.
- Regulatory Environment: Lack of consistent regulations required for AI applications in industry that could make adoption uncertain.
Cost Implications
- Expensive Initial Outlay: Introducing hardware, software or a team with capabilities to implement deep learning can be expensive, and especially resource-intensive for smaller companies.
- Continuing costs: The operation and remodeling of AI systems take ongoing expenses, such as data expense, the cost of updating algorithms and infrastructure etc.
In spite of these obstacles, if strategically mitigated they can reveal additional potential of deep learning for improving velocity models. Through collaboration, innovation, and ethical behavior, institutions and industries can address these challenges and harness the disruptive potential of AI in geophysics. Semblance features deep learning velocity model
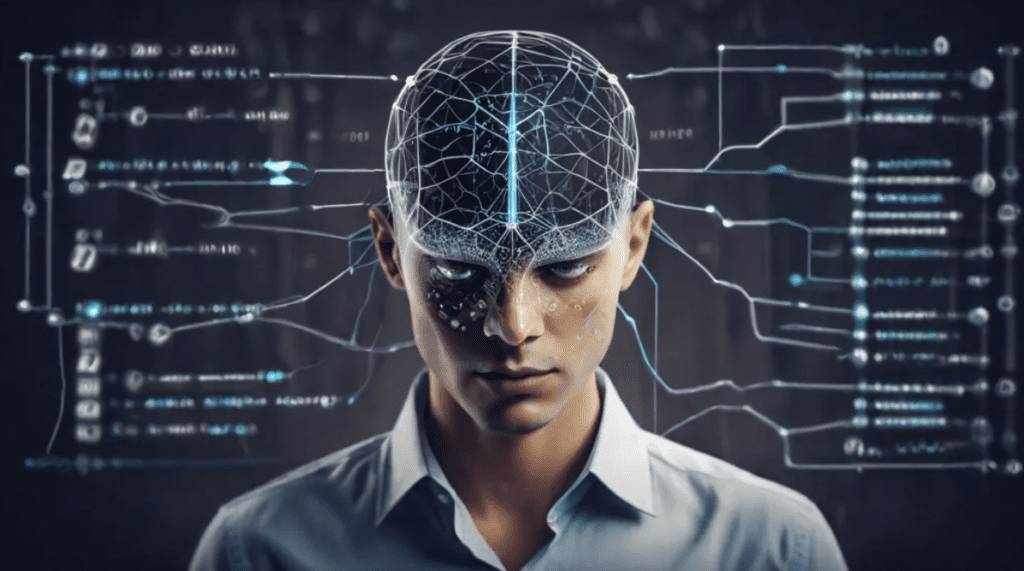
What are possible solutions to these problems?
Surmounting the difficulties of applying deep learning to the velocity model accuracy problem is not a straightforward process, but rather requires a coordinated effort. The following are possible interventions for overcoming these challenges: Some possible solutions to overcome these barriers are as the following:
Enhancing Quality and Accessibility of Data
- Noise Reduction Methods: Use sophisticated signal processing methods (e.g. filtering, and de-noising algorithms) to improve the quality of seismic records.
- Synthetic Data Generation: exploit generated seismic data to fill the gap for real-world data, in particular if not labeled data are available.
- Crowdsourcing Labels: Employ domain experts or use crowdsourcing platforms to annotate the seismic data to develop a quality training data set.
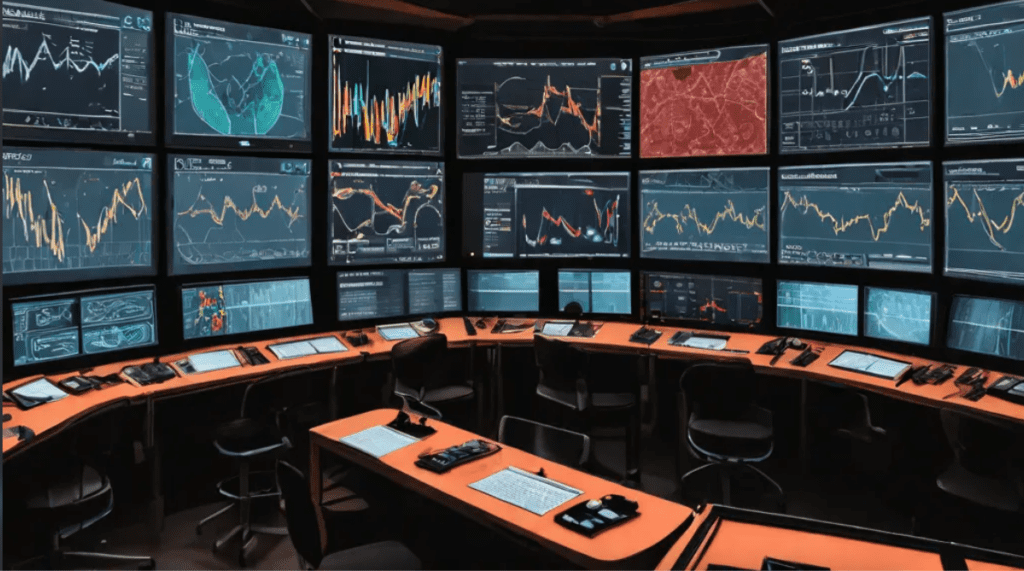
Computational Limitations Problem
- Cloud Computing: Leverage cloud platforms to provision scalable computing resources and avoid investment in expensive on-premise infrastructure.
- Model Optimization: Employ methods such as model pruning, quantization to make the model run on relatively low-computational resources without loss of performance.
- GPU Usage: Maximise the potential of your GPUs and TPUs (tensor processing units) for deep learning calculations.
Improvement on the Performance of the Algorithm
- Explainable AI (XAI): Create models that are easy to understand for humans in order to improve transparency and confidence in ML and AI models.
- Regularization: Try dropout or weight decay to prevent overfitting and improve generalizability.
- Mitigation of Bias: Use a modular training set and fairness of algorithm in an effort to reduce bias in velocity model predictions.
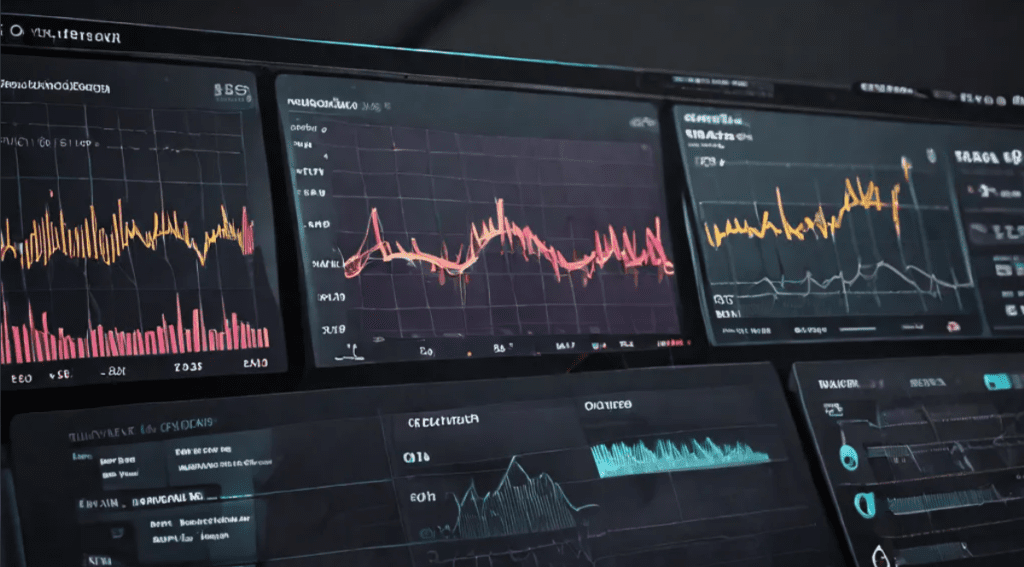
Bridging the Skill Gap
- Training offerings: Contribute to workshops and certification trainings to educate geophysicists on AI and deep learning.
- Collaborative Research: Foster collaborations between the Academic, AI researchers and industry experts to interchange the knowledge.
- User friendly tools: Design software with easy to use interfaces, allowing geophysicists to leverage deep learning without being a computer science major.
Seamless Workflow Integration
- Bespoke AI solutions: Create AI products that are personalised for the special needs of incumbent seismic workflows to enable compatibility and adoption.
- Rolling out the tech: Walk, don’t run, on deep learning systems, starting with low-risk tasks (like velocity picking) before tackling more complex operations.
- Read More Real-time Processing: System to process on the fly and update veloc-ity models in real time during seismic acquisition.
Ethical and Regulatory Contexts
- Data Security: Develop strong data encryption techniques to protect the privacy and security of sensitive seismic data.
- Ethics: Defining industry policy on the ethical use of AI in geophysics including, for example, the need for transparency and accountability.
- Regulatory Advocacy: You’ll collaborate with policymakers to design clear regulations that enable safe and effective deployment of AI.
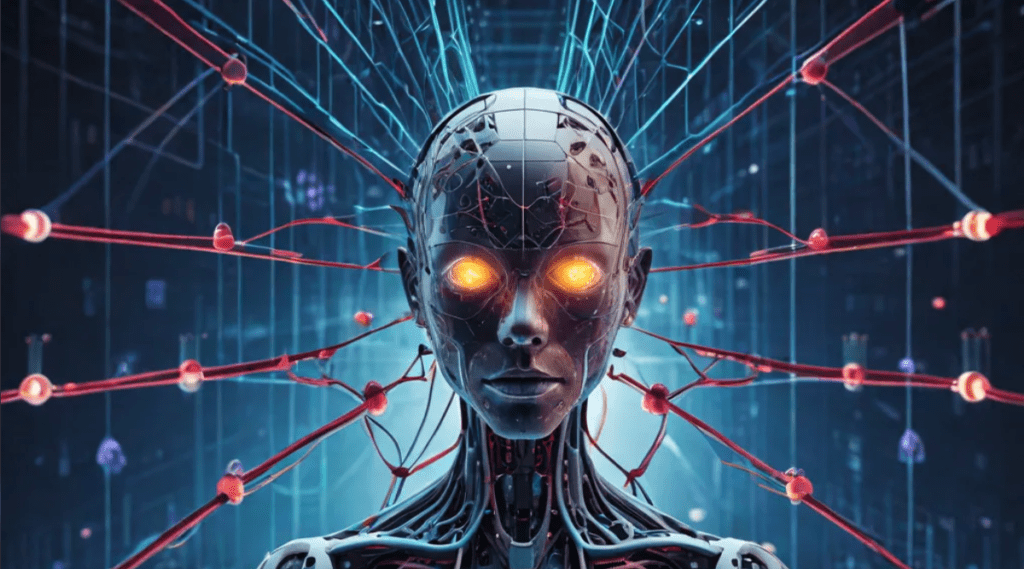
Cost Management Strategies
- Collaborative Funding: Share the effort with other bodies and share the cost of ‘doing’ deep learning by creating a fund to which organisations can contribute.
- Open-source tools: Take advantage of open-source AI tools and libraries for minimized software expenses.
- ROI over the long run: Stress on the long-term gains like efficiency and accuracy to justify the initially sunk-in costs.
By addressing these challenges via novel approaches, the geophysics community can harness the full potential of deep learning for improved velocity models. Not only do these strategies overcome the immediate challenges, they enable a sustainable and scalable implementation.
Conclusion
The combination of semblance features and deep learning is an innovation in seismic data analysis and velocity model building. Through seismic imaging, the researchers demonstrate that they can obtain the quality of the image as good as traditional method using only 3% of the data that is traditionally used. In times to come, as technology develops further, the new kind of applications of such an approach will provide room for interesting findings and development in this area.
This blog has covered how semblance attributes and deep learning have revolutionized velocity model accuracy, revealing the advantages, uses, and possibilities of both technologies. By adopting these annovattions, the geophysics community will open up new possibilities, leading to a The potential for Geophysical discovery to solve many industrial and societal problems).
Also read about Semblance analysis.
Also read about The Future of AI in Middle East